Episodes
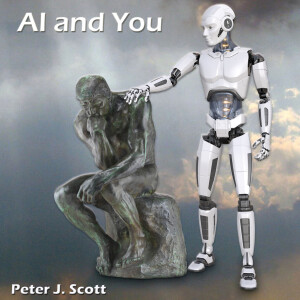
Monday Nov 22, 2021
075 - Guest: Michael Hind, IBM AI Explainability Expert, part 2
Monday Nov 22, 2021
Monday Nov 22, 2021
This and all episodes at: https://aiandyou.net/ .
Training an AI to render accurate decisions for important questions can be useless and dangerous if it cannot tell you why it made those decisions. Enter explainability, a term so new that it isn't in spellcheckers but is critical to the successful future of AI in critical applications. Michael Hind is a Distinguished Research Staff Member in the IBM In part 2, we talk about the Teaching Explainable Decisions project, some of Michael’s experience with Watson, the difference between transparency and explainability, and a lot more. All this plus our usual look at today's AI headlines. Transcript and URLs referenced at HumanCusp Blog. |
![]() |
Comments (0)
To leave or reply to comments, please download free Podbean or
No Comments
To leave or reply to comments,
please download free Podbean App.